How to Handle Missing Data
As anyone who has ever tried to compile data can tell you, missing data is problematic in large part because it decreases a sample’s ability to represent a larger population. When a few pieces of data are lost or corrupted, the validity of the analyses and conclusions are fundamentally threatened. In this post, I’ll outline the most common reasons for missing data and how to handle missing data when it happens.
Reasons for Missing Data
Data can be missing for a number of reasons. Poor research design, non-response or refusal from respondents, and natural attrition can all play a role in data being missing. Whatever the reason may be, it is imperative for researchers to identify the reason for missing data and to understand any patterns in the data that is missing. There are three industry-standard classifications of missing data that researchers should report to consumers of the data set or analysis (Saunders, et al., 2006):
- Missing Values Completely at Random (MCAR) – MCAR refers to situations where the probability that values of one variable are missing is completely unrelated to any other variable in the study.
- Missing at Random (MAR) – MAR refers to missing values that are not related to the outcome being measured, but could be related to at least one other variable in the data set or analysis.
- Missing Not at Random (MNAR) – MNAR is the most frequently used classification for missing data. This classification refers to missing values that have a systematic pattern that are related to at least one of the measured outcomes.
Methods of Addressing Missing Data
- Pairwise Deletion – Also known as available-case analysis, pairwise deletion is the technique of performing analysis on available values, essentially ignoring missing values. For example, a researcher may collect responses via a mailed survey in which some respondents chose not to write in a response for certain questions. The researcher would implement pairwise deletion by simply ignoring the omitted responses and only analyzing the available responses. This will cause sample sizes to vary between variables that have missing values vs. those that do not(Statistics Solutions, n.d.).
- Listwise Deletion – Also known as complete-case analysis, listwise deletion removes all data for a case that has one or more missing values. Only records that have values for all variables are used in analysis for all variables. Continuing with the example above, if a respondent of a mailed survey chose not to answer one of the questions in the survey, the researcher would implement listwise deletion by not including any of the respondent’s responses in the analysis. The researcher would delete the respondent’s record from the data because they did not answer all of the questions. (Statistics Solutions, n.d.).
- Imputation – This is a general term used for any method in which a missing value is replaced with a value derived from some method of imputation. Methods of imputation vary widely in approach and sophistication as well as in their advantages and disadvantages. In our mail survey example, questions that respondents chose not to answer would require the researcher to somehow infer what a respondent would have responded based on either the responses of other respondents or the respondent’s responses to other questions. This process of inferring missing values is the essence of imputation. Methods such as mean imputation, where the mean of the observed values of a variable is used to replace missing values, and regression imputation, where a regression model based on the other, non-missing variables is used to predict the missing values, are some of the most basic and commonly used methods(Groves, et al., 2009).
To Impute or Not to Impute?
The answer to this question should be dictated largely by the reason the data is missing, the missing value classification and the effect that the missing data has on confidence intervals and statistical power. Generally, pairwise deletion and listwise deletion are only used in handling missing values classified as MCAR as imputation is usually unnecessary (Statistics Solutions, n.d.). Methods of imputation will vary in the criteria that calls for their use, but in any case the key for researchers is to fully understand the effect that imputation will have on the distributions of their data and the statistical power of the data set (Groves, et al., 2009).
Conclusion
This provides a summary of the generally accepted classifications of and methods for dealing with missing data. A variety of additional methods exist with their own nuances that dictate their use, however this overview will serve any researcher well in understanding the basics of working with missing values in their data sets. It is important to note that in many cases, researchers can design studies so that they effectively limit the risk of having missing data in their data sets. As Dr. Paul Allison, a professor of sociology and well-known expert in the field of statistical methods says, “The only really good solution to the missing data problem is not to have any. So in the design and execution of research projects, it is essential to put great effort into minimizing the occurrence of missing data. Statistical adjustments can never make up for sloppy research (Allison, 2001).”
References
Allison, P. D. (2001). Multiple Imputation for Missing Data: A Cautionary Tale. Retrieved December 6, 2015
Groves, R. M., Fowler, Jr., F. J., Couper, M. P., Lepkowski, J. M., Singer, E., & Tourangean, R. (2009). Survey Methodology (2nd ed.). Hoboken, New Jersey: John Wiley & Sons, Inc.
Saunders, J. A., Morrow-Howell, N., Spitznagel, E., Dore, P., Proctor, E. K., & Pescarino, R. (2006). Imputing Missing Data: A Comparison of Methods for Social Work Researchers. National Association of Social Workers, 20. Retrieved December 4, 2015, from https://cmhsr.wustl.edu/Resources/Documents/Imputing%20missing%20data_A%20comparison%20of%20methods%20for%20Social%20Work%20Researchers.pdf
Soley-Bori, M. (2013, May 6). Dealing with missing data: Key assumptions and methods for applied analysis. 4. Retrieved December 5, 2015, from https://www.bu.edu/sph/files/2014/05/Marina-tech-report.pdf
Statistics Solutions. (n.d.). Missing Data: Listwise vs. Pairwise. Retrieved December 4, 2015, from Statistics Solutions: https://www.statisticssolutions.com/missing-data-listwise-vs-pairwise/
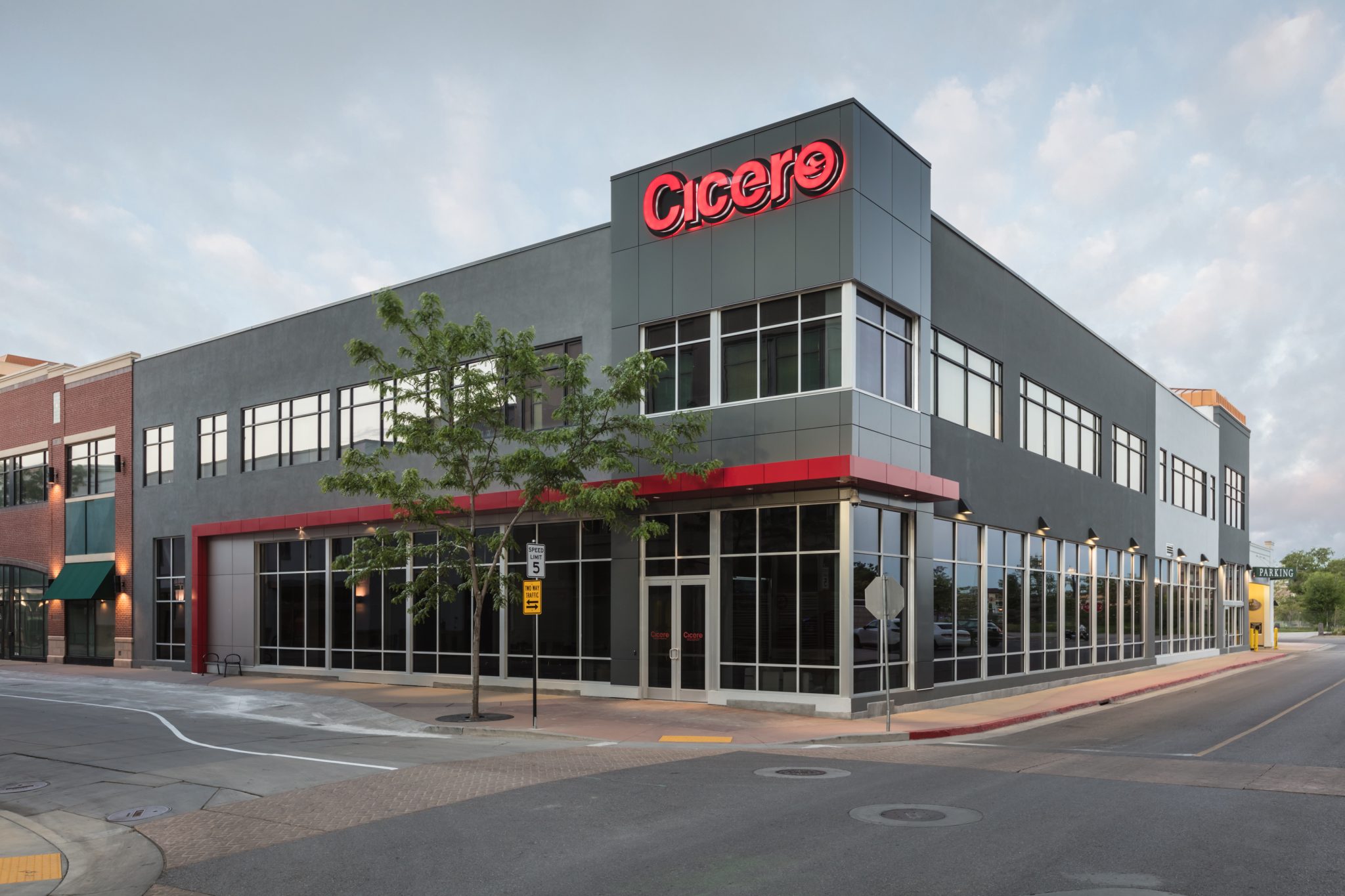
Start a Conversation
Thank you for your interest in Cicero Group. Please select from the options below to get in touch with us.